Bragadeesh Sundararajan, Chief Data Science Officer, Dvara KGFS shares the data lake journey of his organization:
Ravi Lalwani: What is the data lake journey of Dvara KGFS and what were the key milestones?
Bragadeesh Sundararajan: A data lake journey is a process of storing, transforming, and analyzing large amounts of data in a central repository known as a data lake. Our journey involved the following steps:
Data Ingestion: This is the process of collecting and storing data from various transactional databases. Data is ingested in real-time or batch mode, depending on the requirements of the organization.
Data Storage: Once the data has been ingested, it needs to be stored in a central repository. A data lake is a type of repository that can store structured and unstructured data in its raw form, allowing it to be accessed and analyzed later. We are using MySQL as the central data repository for all data ingestions.
Data Transformation: After the data has been stored in the data lake, it typically needs to be transformed and cleaned before it can be analyzed. This may involve processes such as filtering, aggregating, and joining data from different sources. Python scripts are used to transform the data and store it in cleaned data.
Data Analysis: Once the data has been transformed, it can be analyzed using a variety of tools and techniques, such as SQL queries, machine learning algorithms, or visualization tools. The results of this analysis can be used to gain insights, make decisions, and drive business value. Python scripts are used for ML, AI, and data analytics.
Data Visualization: The results of the data analysis can be presented in a variety of ways, such as dashboards, reports, or visualizations, to help stakeholders understand and make use of the insights gained from the data. We use open source business intelligence tool ‘Metabase’ for data visualization & reporting.
What are the top 3 business applications of the data lake for your organization?
We got benefited by using data lakes to support a variety of our business applications. Here are 3 examples:
Credit Risk Assessment: A data lake can be used to store and analyze data from various sources, such as credit bureau data, transactional data, and demographic data, to assess the credit risk of potential borrowers. Machine learning algorithms can be used to analyze the data and predict the likelihood of default.
Customer Segmentation & Targeting: A data lake can be used to store and analyze customer data from various sources, such as transactional data, social media data, and marketing data. This can be used to segment customers into different groups and tailor marketing campaigns and product offerings to specific segments.
Customer Default Prediction: This is the process of predicting the likelihood of a customer defaulting on a loan or credit card payment. This can be important for financial institutions, as defaulting customers can result in significant losses. There are several approaches to customer default prediction, including statistical modelling and machine learning. Statistical modelling involves using statistical techniques, such as logistic regression, to build a model that predicts the probability of default based on a set of input variables. Machine learning involves training a model on a large dataset of customer data and using the model to predict the likelihood of default. Several factors can influence the probability of default, such as the customer’s credit score, income, and debt-to-income ratio. Other factors, such as the customer’s age, education level, and employment status, can also be important.
What is the size of the data lake and at what rate is it growing? What kinds of data are not going into the data lake? What is the reason?
The total size of our CDR is approximately 1.3 TB with 10-12% data growth year on year (for the size and growth). There is no specific type of data that is inherently excluded from a data lake. Data lakes are designed to store large amounts of data in their raw form and can accommodate structured, unstructured, and semi-structured data from a wide variety of sources.
However, there may be certain types of data that an organization decides not to store in its data lake for various reasons. We have chosen not to store sensitive or personal data in a data lake due to privacy concerns. Data that is not relevant to the organization’s business objectives may also be excluded from the data lake to avoid cluttering the repository and making it more difficult to find relevant data. In addition, data lakes may not be the most suitable repository for certain types of data that require low latency or high transaction processing, as data lakes are optimized for batch processing and may not offer the same level of performance as a traditional database. In these cases, it may be more appropriate to store the data in a different repository that is better suited to the specific requirements of the data.
Is the data lake in the public cloud or on-prem? If on-prem, is it a private cloud? Who are the key technology and consulting partners for the data lake?
Our data lake is on the Google public cloud (GCP). There are several technologies and consulting firms that offer products and services related to data lakes. These can include hardware and software vendors, as well as consulting firms that specialize in helping organizations design and implement data lake solutions. Ours is on-prem MySQL DB and built on our own without any consulting partner.
____________
Read more-
Experiential Digital Done Right
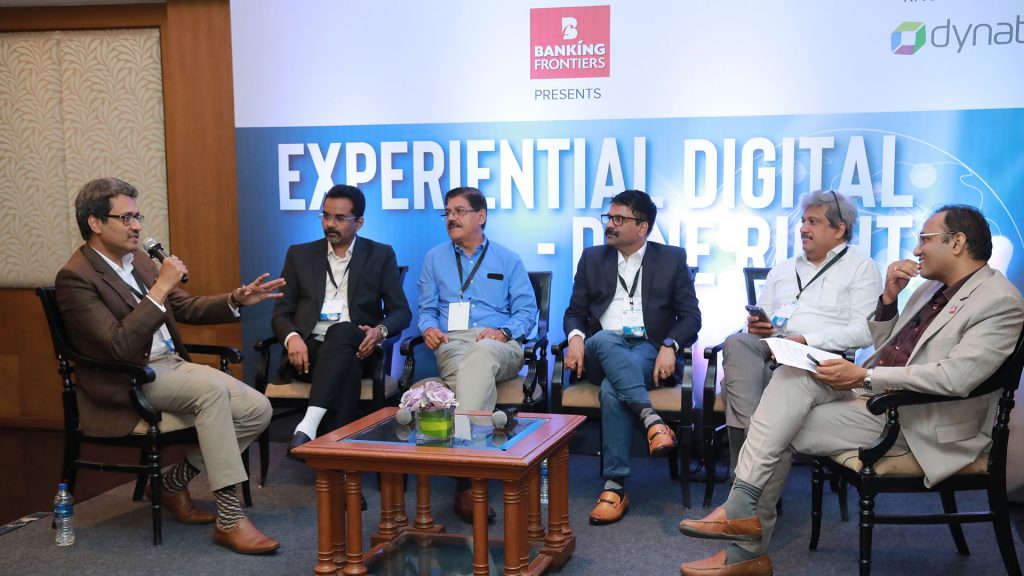
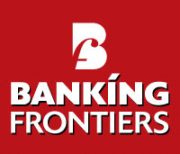