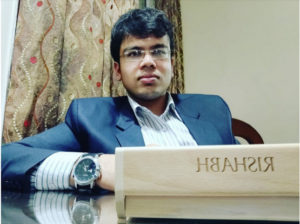
Rishabh Goel , Co-founder and CEO, Credgenics
Across the globe, every country’s banking sector is responsible for empowering its economic and social development. Banks collect funds together from customers and make them available for investments via issuing loans, thereby providing liquidity and allowing smooth flow of savings and investments. In emerging economies, banks are more than mere enablers of financial intermediation and support the additional responsibility of making up for the nation’s social agenda.
The key challenge going forward for Indian banks and NBFCs is to expand credit portfolio and effectively manage NPAs while maintaining profitability. Asset quality continues to be the biggest challenge for financial institutions in the present dynamic environment. The issue of rising NPAs in India has put the entire banking and lending market under an enormous amount of pressure. According to the Reserve Bank of India’s Financial Stability Report of June 2019, the gross NPA ratio of the scheduled commercial banks rose from 10.2% in September 2018 to 11.6% in March 2019. This scenario may further worsen due to the PCA framework that could help mitigate financial stability risks.
Artificial intelligence (AI) is a recent technology enabler which refers to the human brain simulation by machines to carry out functions like logical reasoning, learning, self-correction, robotics etc. Big Data Analytics refers to the extraction of relevant insights from data using various techniques from fields like machine learning, computer programming, statistical modelling, pattern recognition, data warehousing, cloud computing etc. to discover hidden patterns, unknown correlations, current market trends, basic customer preferences and other useful information that can help organizations make more-informed and profitable business decisions.
The key to use AI and reduce bad loans is twofold – first improving the underwriting mechanisms and second strengthening the collections processes. Technology can add tremendous weightage to both these legs:
Underwriting: Lenders need to have all the relevant data about the borrowers to make an informed decision to process or extend credit line. Data analytics is the main key to the solution. It will help NBFCs and banks gather and categorize the borrower thereby assigning a risk score to each one of them. This can be implemented for 2 aspects – verification of new loan applications and continuous monitoring of existing borrowers. Drawing inferences from the previous attempts of data processing, technology is more matured today and can provide concrete solutions to effectively identify stressed accounts, extract borrowers’ social media profiles and classify loan account health, based on heaps of available borrower data.
Collections & Recovery: Recovery of NPAs is a time-consuming process that requires deploying and managing new resources that could increase the underlying costs burden on the financial institutions. Here’s where a blend of technology and taskforce could help scale up the productivity and speed up the collection process to tackle the problem of NPAs. The systemic approach could greatly work at the root level to identify stressed accounts based on the set parameters of the banks and notify the taskforce which can focus on recovery strategy and collaboration for resolution. Entire workflow can be automated and digitized to a greater extent in order to remove scope of manual intervention. Moreover, these improvements in analytics and automation of processes could deliver on quick & transparent decision making. This will bring about greater accountability of financial institutions, effective monitoring of borrowers and prioritization of stress accounts.
Risk Management
Banks are considering machine learning to make sense of large, unstructured and semi-structured datasets and to police the outputs of primary models. This will help in proper evaluation of credit proposals, based on risk rating and hence prevent accounts from conversion into NPAs.
The major factors that affect NPAs at the bank-level include:
- Improper Due Diligence
- Lenient Credit terms
- Loose Credit Monitoring
- Collateral free loans given
- Frauds
- Wilful default by customers
All these factors responsible for surge in NPAs can be addressed and controlled by AI and Big Data Analytics. Machine learning can be applied to systemic risk identification, assessment and propagation channels. Specifically, NLP tools may help authorities to detect measure, predict, and anticipate, among other things, market volatility, liquidity risks, financial stress, housing prices, and unemployment.
While the concept of AI has been around for decades, it is only recently that the AI fantasy has started to turn into reality. Many of the technology pieces are already in place, in varying stages of maturity. Many banks have made a start by incorporating several AI components into their processes and have experienced early results. As we have seen in this paper, most of the issues of handling NPA management can be handled using AI and Big Data Analytics, which will help in strengthening balance sheets of banks and further enhance credit quality. In global context, many banks have already started using AI and data analytics for risk management and fraud detection, which has not only reduced the level of rising NPAs but also improved identification of creditworthy customers to create a foundation of a good loan profile.
Applications of AI and machine learning may enhance the interconnectedness of financial markets and institutions in unexpected ways. Institutions’ ability to make use of big data from new sources may lead to greater dependencies on previously unrelated macroeconomic variables and financial market prices, including from various non-financial corporate sectors (e-commerce, sharing economy, etc.). As institutions find algorithms that generate uncorrelated profits or returns, there is a risk these will be exploited on a sufficiently wide scale that correlations actually increase. These potentially unforeseen interconnections will only become clear as technologies are actually adopted.
Rishabh Goel is co-founder and CEO of Credgenics
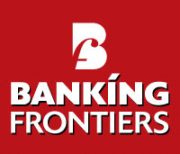